How AI is revolutionizing healthcare operations
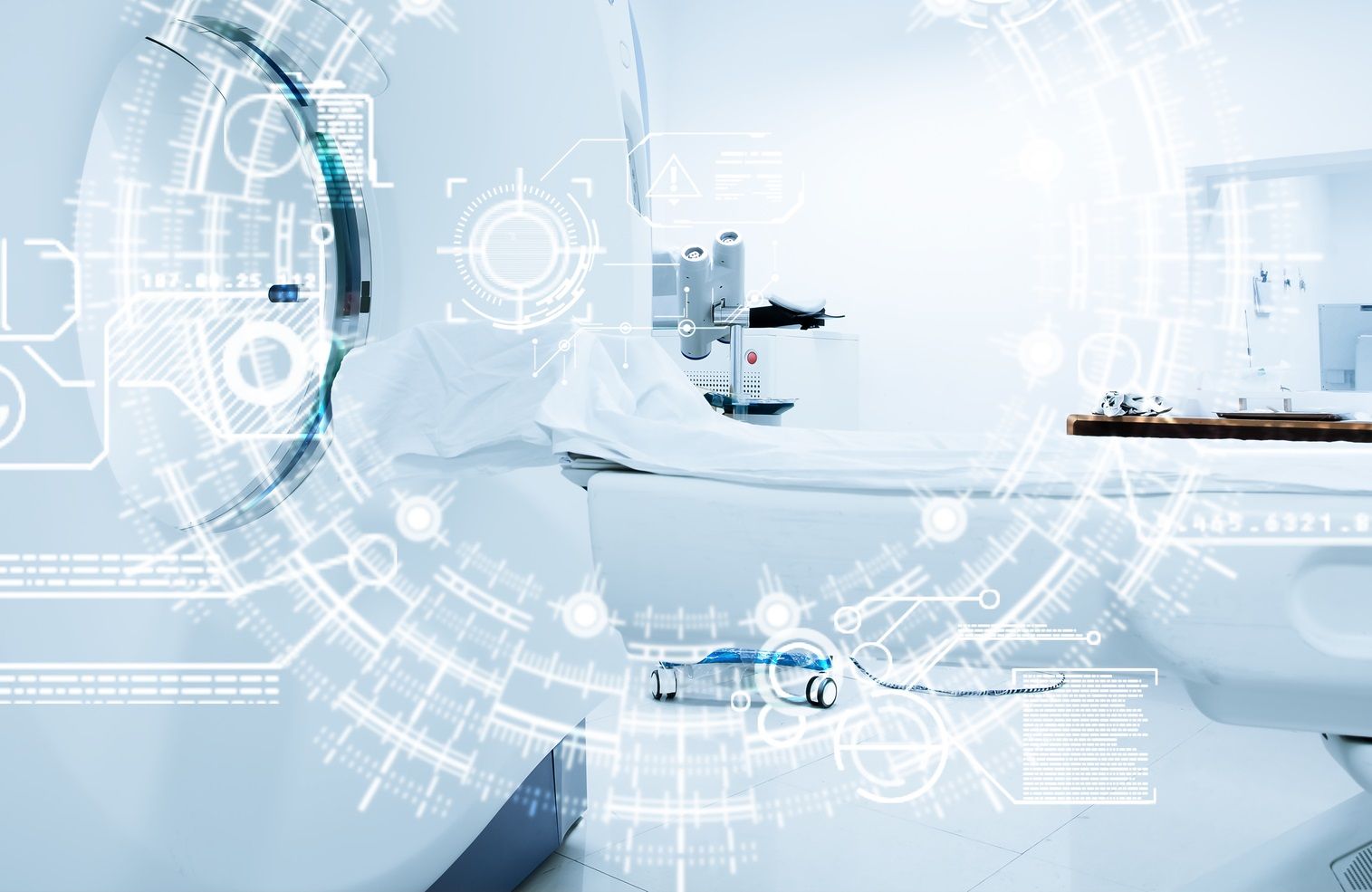
By: Paul Bergstrom, EVP Head of Global Services at Elekta
Artificial intelligence (AI) is reshaping operations across industries. Arguably, healthcare is where these changes are poised to make the biggest impact – optimizing uptime and availability of the treatment solutions. The potential of AI in healthcare plays out in growth predictions. In 2014, the national AI healthcare market was worth $600 million. By 2021, the figure will rise to $6.6 billion, according to a 2018 report from Accenture.
For an industry facing high costs, the technology could also mean big savings, as administrative and operational inefficiencies account for nearly one third of the U.S. healthcare system’s $3 trillion in annual costs. Using AI-powered tools capable of processing large amounts of data and making real-time recommendations, healthcare organizations are learning they can reduce administrative waste in a number of areas, from medical equipment maintenance to hospital bed assignments.
Seeing the potential in these new technologies, investing in big data and AI is a rising priority among healthcare executives. According to a 2019 survey, 77 percent of healthcare executives reported that their organizations are accelerating their investments in AI, citing business transformation, agility and competition as principal drivers.
What do we mean when we say “AI”?
Artificial intelligence is reinventing and reinvigorating modern healthcare through technologies that can predict, comprehend, learn and act. The ability of AI to transform clinical care has received widespread attention, but the technology’s potential extends beyond patient care to processes across the spectrum of healthcare operations. There is a lot being published on AI, machine learning and deep learning. It is helpful to understand the relationship between these three concepts to better understand how they are being used. What has made all levels possible is the amount of data that is now available today and the computing power to process it.
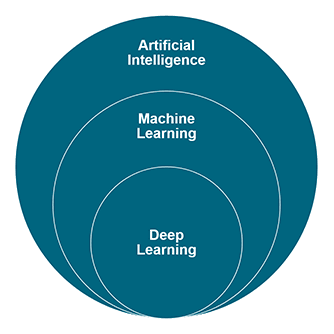
Artificial Intelligence – when a machine can complete a task based on a set of rules to solve a problem.
Machine Learning – a subset of AI where the algorithm is provided with data characteristics so it can begin to “learn” and predict based on these defined characteristics.
Deep Learning – a subset of machine learning where the specific data characteristics are not defined and the algorithms “learn” what criteria is needed to categorize data elements and then predict.
Medical technology and medical device manufacturers are beginning to implement predictive maintenance approaches to help remotely monitor equipment and predict failures before any disruption occurs. This enables systems to be repaired remotely or at a time that is convenient for the users, reducing operational downtime.
If you can predict it, you can prevent it
In healthcare and other industries that depend on reliable equipment performance, few things are more disruptive than unexpected outages. These unplanned stops create costly emergency situations, such as extended downtime, rush delivery of parts and overtime to repair the equipment. Equally important are an array of esoteric cost factors that involve safety, waste and even brand. In fact, the International Society of Automation estimates that manufacturers globally lose $647 billion to machine downtime each year.
Facing pressure to improve profitability and efficiency, many healthcare organizations are turning to emerging technologies like AI and big data analytics to improve upon existing maintenance operations. Until recently, maintenance typically involved either reacting to an unexpected problem or adhering to a preventive maintenance schedule, which can sometimes result in unnecessary maintenance.
Machine learning (ML) and Deep learning (DL) provide the opportunity to process massive amounts of data faster than ever before. By leveraging ML and DL models to identify patterns, the information derived from equipment sensors can be turned into meaningful and actionable insights for proactive maintenance of assets, preventing incidents that result in unplanned clinical downtime. Known as predictive maintenance, this added intelligence enables organizations to forecast when or if equipment will fail so that its maintenance and repair can be scheduled before the failure occurs.
Proactive support and predictive maintenance with Elekta IntelliMax
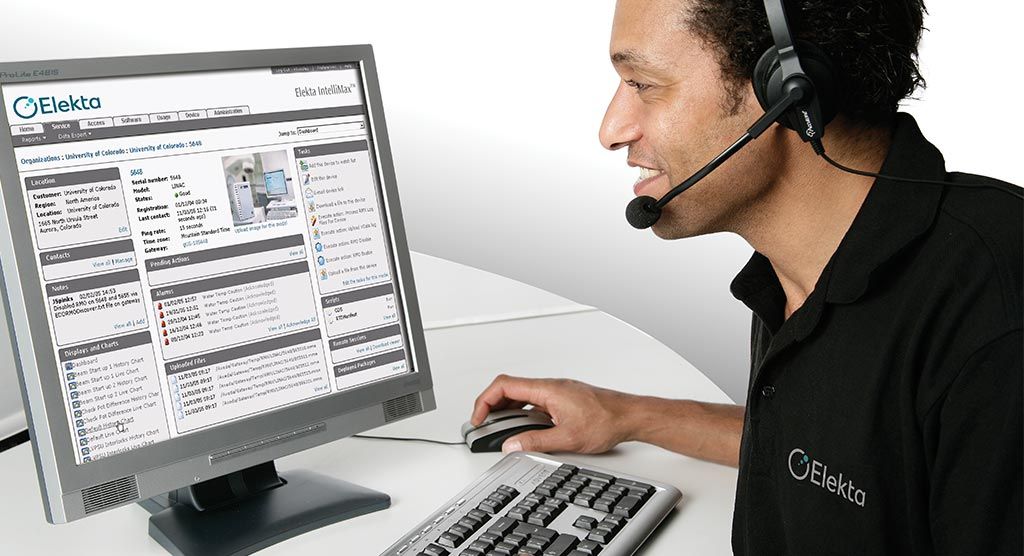
At Elekta, big data, AI and other digital tools offer new capabilities that can improve service and support. For instance, over 80 percent of Elekta’s global-installed base of linear accelerators are connected to Elekta IntelliMax®, our remote system for proactive support and predictive maintenance.
IntelliMax enables a service engineer to see and resolve potential problems before they arise, maximizing clinical availability, minimizing costs and reducing the chance of disruption for the patient. This year, more than 3,000 IntelliMax-generated cases have been raised to avoid clinical interruptions and downtime. 75 percent of these predictive cases were raised through the AI algorithms, and the percentage is rapidly increasing over time, thanks to a continuously improving AI model.